AI Tools Can’t Revolutionise Public Health if They Stick to Old Patterns
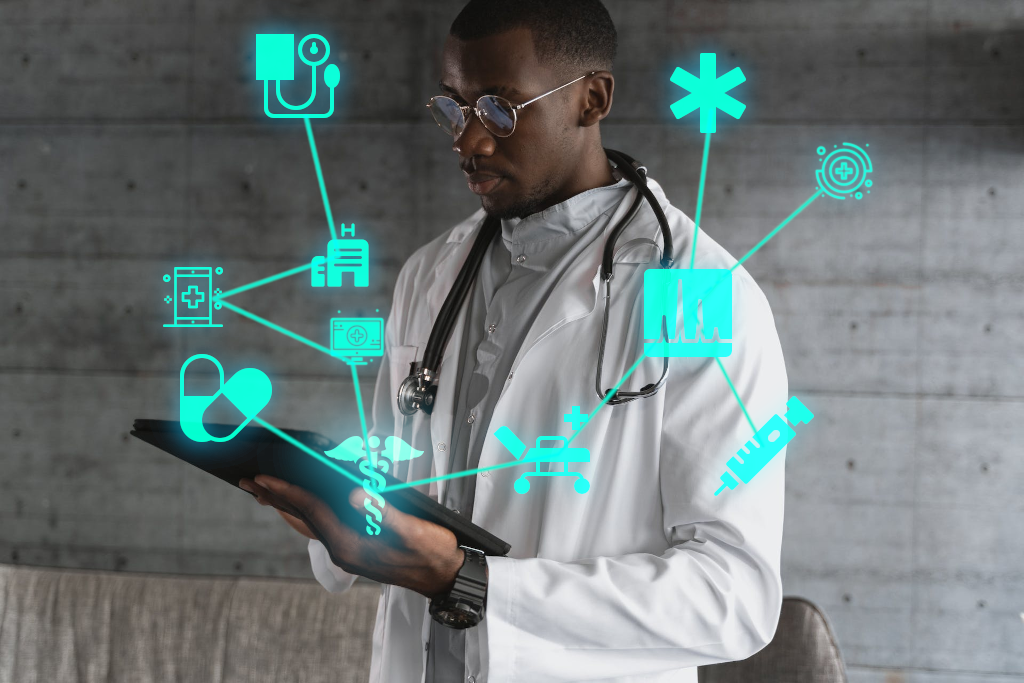
As tools powered by artificial intelligence increasingly make their way into health care, the latest research from UC Santa Cruz Politics Department doctoral candidate Lucia Vitale takes stock of the current landscape of promises and anxieties.
Proponents of AI envision the technology helping to manage health care supply chains, monitor disease outbreaks, make diagnoses, interpret medical images, and even reduce equity gaps in access to care by compensating for healthcare worker shortages. But others are sounding the alarm about issues like privacy rights, racial and gender biases in models, lack of transparency in AI decision-making processes that could lead to patient care mistakes, and even the potential for insurance companies to use AI to discriminate against people with poor health.
Which types of impacts these tools ultimately have will depend upon the manner in which they are developed and deployed. In a paper for the journal Social Science & Medicine, Vitale and her coauthor, University of British Columbia doctoral candidate Leah Shipton, conducted an extensive literature analysis of AI’s current trajectory in health care. They argue that AI is positioned to become the latest in a long line of technological advances that ultimately have limited impact because they engage in a “politics of avoidance” that diverts attention away from, or even worsens, more fundamental structural problems in global public health.
For example, like many technological interventions of the past, most AI being developed for health focuses on treating disease, while ignoring the underlying determinants of health. Vitale and Shipton fear that the hype over unproven AI tools could distract from the urgent need to implement low-tech but evidence-based holistic interventions, like community health workers and harm reduction programs.
“We have seen this pattern before,” Vitale said. “We keep investing in these tech silver bullets that fail to actually change public health because they’re not dealing with the deeply rooted political and social determinants of health, which can range from things like health policy priorities to access to healthy foods and a safe place to live.”
AI is also likely to continue or exacerbate patterns of harm and exploitation that have historically been common in the biopharmaceutical industry. One example discussed in the paper is that the ownership of and profit from AI is currently concentrated in high-income countries, while low- to middle-income countries with weak regulations may be targeted for data extraction or experimentation with the deployment of potentially risky new technologies.
The paper also predicts that lax regulatory approaches to AI will continue the prioritization of intellectual property rights and industry incentives over equitable and affordable public access to new treatments and tools. And since corporate profit motives will continue to drive product development, AI companies are also likely to follow the health technology sector’s long-term trend of overlooking the needs of the world’s poorest people when deciding which issues to target for investment in research and development.
However, Vitale and Shipton did identify a bright spot. AI could potentially break the mold and create a deeper impact by focusing on improving the health care system itself. AI could be used to allocate resources more efficiently across hospitals and for more effective patient triage. Diagnostic tools could improve the efficiency and expand the capabilities of general practitioners in small rural hospitals without specialists. AI could even provide some basic yet essential health services to fill labor and specialization gaps, like providing prenatal check-ups in areas with growing maternity care deserts.
All of these applications could potentially result in more equitable access to care. But that result is far from guaranteed. Depending on how and where these technologies are deployed, they could either successfully backfill gaps in care where there are genuine health worker shortages or lead to unemployment or precarious gig work for existing health care workers. And unless the underlying causes of health care worker shortages are addressed – including burnout and “brain drain” to high-income countries – AI tools could end up providing diagnosis or outbreak detection that is ultimately not useful because communities still lack the capacity to respond.
To maximise benefits and minimise harms, Vitale and Shipton argue that regulation must be put in place before AI expands further into the health sector. The right safeguards could help to divert AI from following harmful patterns of the past and instead chart a new path that ensures future projects will align with the public interest.
“With AI, we have an opportunity to correct our way of governing new technologies,” Shipton said. “But we need a clear agenda and framework for the ethical governance of AI health technologies through the World Health Organization, major public-private partnerships that fund and deliver health interventions, and countries like the United States, India, and China that host tech companies. Getting that implemented is going to require continued civil society advocacy.”