The Future of Healthcare Interoperability: Building a Stronger Foundation for Data Integration
Henry Adams, Country Manager South Africa, InterSystems
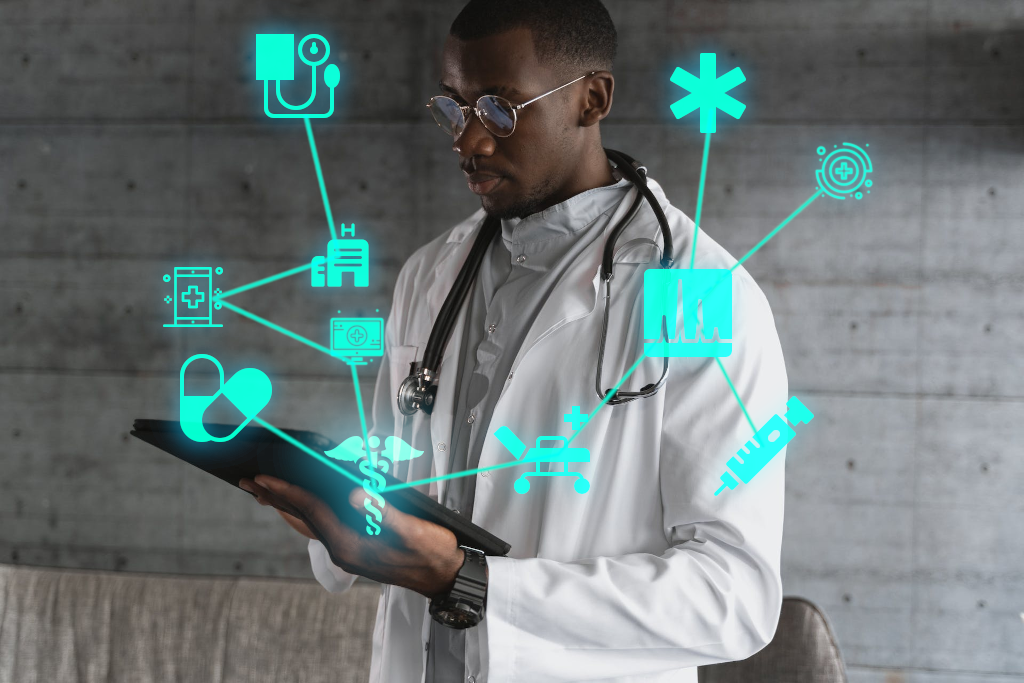
Healthcare data is one of the most complex and valuable assets in the modern world. Yet, despite the wealth of digital health information being generated daily, many organisations still struggle to access, integrate, and use it effectively. The promise of data-driven healthcare – where patient records, research insights, and operational efficiencies seamlessly come together – remains just that: a promise. The challenge lies in interoperability.
For years, healthcare institutions have grappled with fragmented systems, disparate data formats, and evolving regulatory requirements. The question is no longer whether to integrate but how best to do it. Should healthcare providers build, rent, or buy their data integration solutions? Each approach has advantages and trade-offs, but long-term success depends on choosing a solution that balances control, flexibility, and cost-effectiveness.
Why Interoperability Remains a Challenge
Despite significant advancements in standardisation, interoperability remains a persistent challenge in healthcare. A common saying in the industry – “If you’ve seen one HL7 interface, you’ve seen one HL7 interface” – illustrates the lack of uniformity across systems. Even FHIR, the latest interoperability standard, comes with many extensions and custom implementations, leading to inconsistency.
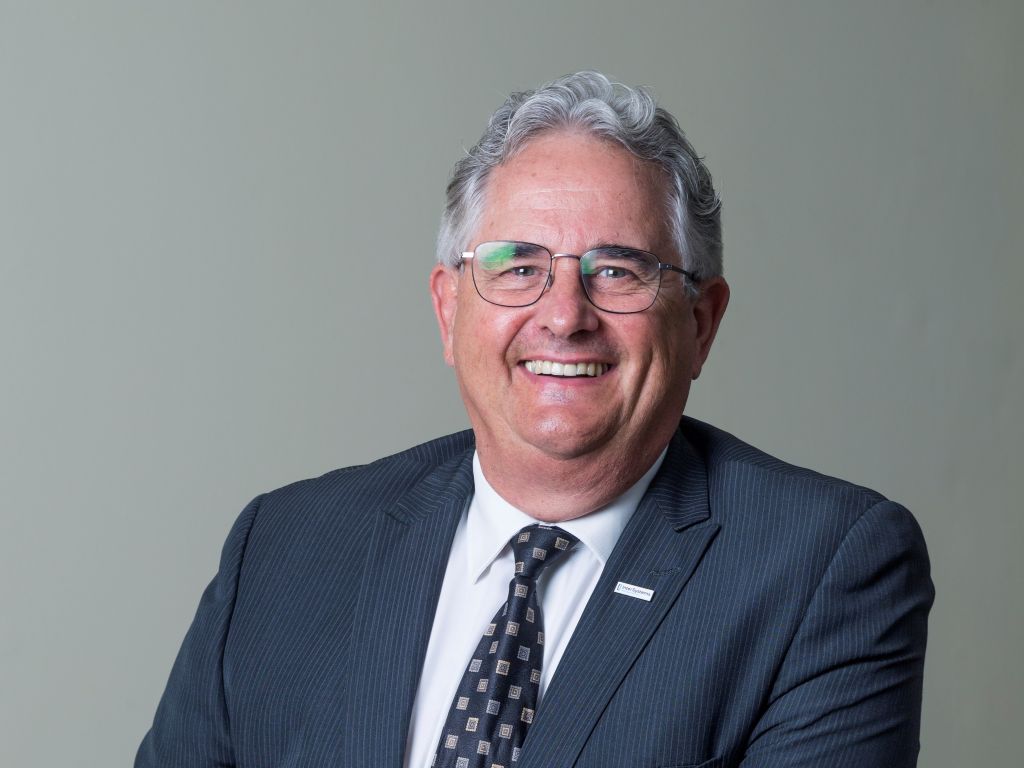
Adding to this complexity, healthcare data must meet strict security, privacy, and compliance requirements. The need for real-time data exchange, analytics, and artificial intelligence (AI) further increases the pressure on organisations to implement robust, scalable, and future-proof integration solutions.
The Build, Rent, or Buy Dilemma
When organisations decide how to approach interoperability, they typically weigh three options:
- Building a solution from scratch offers full control but comes with high development costs, lengthy implementation timelines, and ongoing maintenance challenges. Ensuring compliance with HL7, FHIR, and other regulatory standards requires significant resources and expertise.
- Renting an integration solution provides quick deployment at a lower initial cost but can lead to vendor lock-in, limited flexibility, and escalating costs as data volumes grow. Additionally, outsourced solutions may not prioritise healthcare-specific requirements, creating potential risks for compliance, security, and scalability.
- Buying a purpose-built integration platform strikes a balance between control and flexibility. Solutions like InterSystems Health Connect and InterSystems IRIS for Health offer pre-built interoperability features while allowing organisations to customise and scale their integration as needed.
The Smart Choice: Owning Your Integration Future
To remain agile in an evolving healthcare landscape, organisations must consider the long-term impact of their integration choices. A well-designed interoperability strategy should allow for:
- Customisation without complexity – Organisations should be able to tailor their integration capabilities without having to build from the ground up. This ensures they can adapt to new regulatory requirements and technological advancements.
- Scalability without skyrocketing costs – A robust data platform should enable growth without the exponential cost increases often associated with rented solutions.
- Security and compliance by design – Healthcare providers cannot afford to compromise on data privacy and security. A trusted interoperability partner should offer built-in compliance with international standards.
Some healthcare providers opt for platforms that combine pre-built interoperability with the flexibility to scale and customise as needed. For example, solutions designed to support seamless integration with electronic health records (EHRs), medical devices, and other healthcare systems can offer both operational efficiency and advanced analytics capabilities. The key is selecting an approach that aligns with both current and future needs, ensuring data remains accessible, secure, and actionable.
Preparing for the Future of Healthcare IT
As healthcare systems become more digital, the need for efficient, secure, and adaptable interoperability solutions will only intensify. The right integration strategy can determine whether an organisation thrives or struggles with inefficiencies, rising costs, and regulatory risks.
By choosing an approach that prioritises flexibility, control, and future-readiness, healthcare providers can unlock the full potential of their data – improving patient outcomes, driving operational efficiencies, and enabling innovation at scale.
The question isn’t just whether to build, rent, or buy – but how to create a foundation that ensures long-term success in healthcare interoperability.