Introducing the Future of Personalised Healthcare: Ajuda
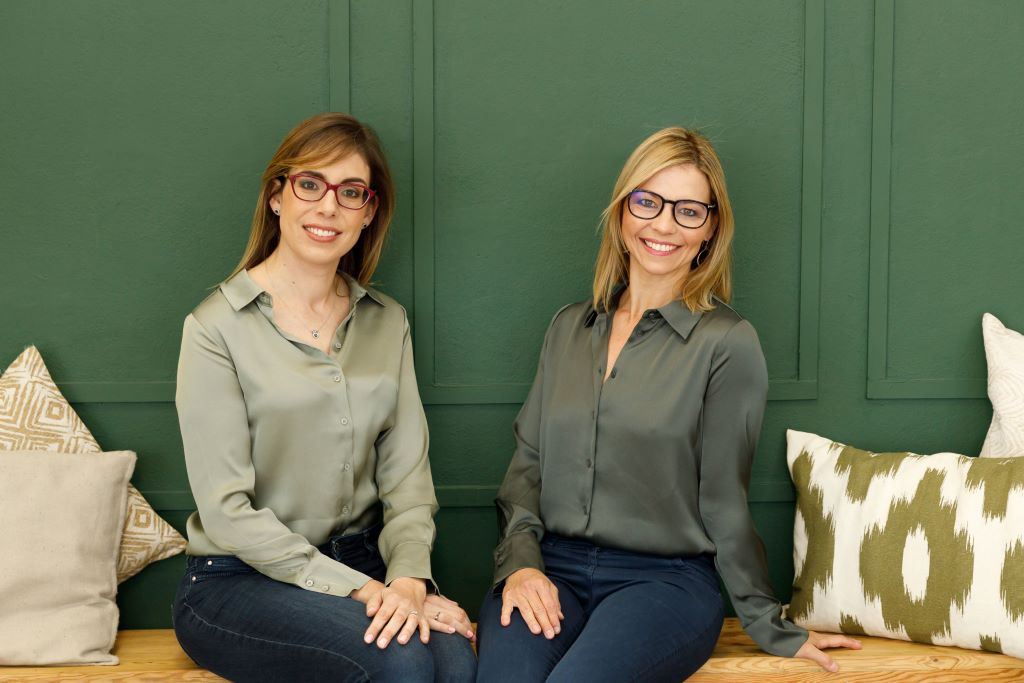
As technology continues to shrink the world, migration is becoming easier. According to Statista, nearly a million South Africans emigrated in 2020, and the semigration trend in recent years has seen hundreds of thousands of South Africans relocating between provinces. While belongings can go into a truck or shipping container, something even more important is slipping through the cracks: personal health records.
Two highly-motivated, entrepreneurial women are looking to change that with an innovative, user-friendly solution – a secure digital health vault called Ajuda, where anyone and everyone can store their health information and get quick access to it anytime, anywhere, using a secure login from their digital device.
Designed With You In Mind
Ajuda (which means ‘help’ in Portuguese) was founded and developed by both Dr Liza Street, a paediatrician, and Taryn Uhlmann, a technology and marketing business executive.
The two – both moms of three – met when Street began treating Uhlmann’s children. After COVID-19, with all the disruptions and innovations that arose during that time, they got chatting about how disempowering it was for moms not to have easy access to their children’s medical records and thus having to rely on memory when it came to their children’s developmental milestones, doctor consults and medication names. “We don’t realise how often we need to recall this information – for new schools, at doctors’ visits and even when relocating. This frustration, especially for busy parents, is where it all started,” says Uhlmann.
That conversation, three years ago, was the seed for what has blossomed into Ajuda. Uhlmann and Street recently welcomed a third member, Allan Sweidan, as an investor and advisor. Sweidan, a clinical psychologist, brings his experience from co-founding Akeso, Netcare’s mental healthcare clinics, and more recently the mental health app, October Health (Panda), to Ajuda.
Why a Central Health Information Vault?
While the idea may have been born out of a conversation about time-strapped moms managing their kids’ health, Uhlmann and Street soon realised that not having the means to securely and conveniently store one’s health information and have access to one’s medical history was a challenge faced by everyone.
Accurate medical records are necessary in emergency situations, anytime you’re having new medication prescribed, for insurance applications, school applications, visa applications, and in many other instances.
What’s more, not having access to personal health information makes it difficult for people to take control of their own health. Having accurate information on hand helps people keep track of which medications to take, in what dosages and when. It also helps healthcare providers make informed decisions around treatments and prescriptions, based on their patients’ health histories, and can lower the risk of adverse drug interactions. In an age of the ‘sandwich generation’, where adults today often take care of both their children and their parents, a centralised repository of their family’s health information is game-changing.
“We looked at all the challenges,” says Uhlmann. “Memory is a big challenge; migration and movement is a big challenge; and the fact that medical care is fragmented – you might have a scan at one hospital and a blood test at another, or maybe you take your child to a GP while you’re on holiday, and in the end, because you don’t have access to all those records in one place, it means no doctor has all the information required to ever look at you holistically. There are many separate medical apps for various healthcare organisations, but no centralised, consumer facing solution pulling it all together. ”
Everything In One Place
Ajuda addresses these concerns with a secure, easy-to use digital storage vault that users can access anywhere, anytime, free of charge.
A second time-saving feature of Ajuda is the ‘One Time Form.’ When signing up, a new user creates a profile for themselves and/or their children, which generates a ‘One Time Form’ and they’re set for life. Creating a profile is simple and interactive, and Ajuda users are guided through the process of completing and uploading their personal and health details, step by step, with user-friendly prompts and explanations.
“This is the same core information that you fill in every time you see a new doctor, have a blood test, or do any medical procedure, which becomes frustrating and time consuming,” says Street. “Now you just need to complete it once, unpressurised, in the comfort of your home, with the correct information at hand, and then take it each time you go to a healthcare provider. It’s a win-win for doctors and patients.”
If users don’t have all their information on hand, no problem – they can fill in the gaps later. Once they’ve completed their profile, they can then enjoy the peace of mind that their personal health information is safely, conveniently and accurately stored.
“For healthcare providers, it provides a comprehensive record of a patient’s health history, not only at their own practice but anywhere the patient has received treatment,” says Street.
Free and Independent
Ajuda is free to use, and you don’t need to be a member of a particular medical scheme or use a particular healthcare provider to access it. By making it free to use, Uhlmann and Street hope to empower everyone with the means to take control of their own health information.
For more information on Ajuda or to sign up, visit Ajuda.co.za