Rising Health Care Prices Result in Non-healthcare Job Cuts
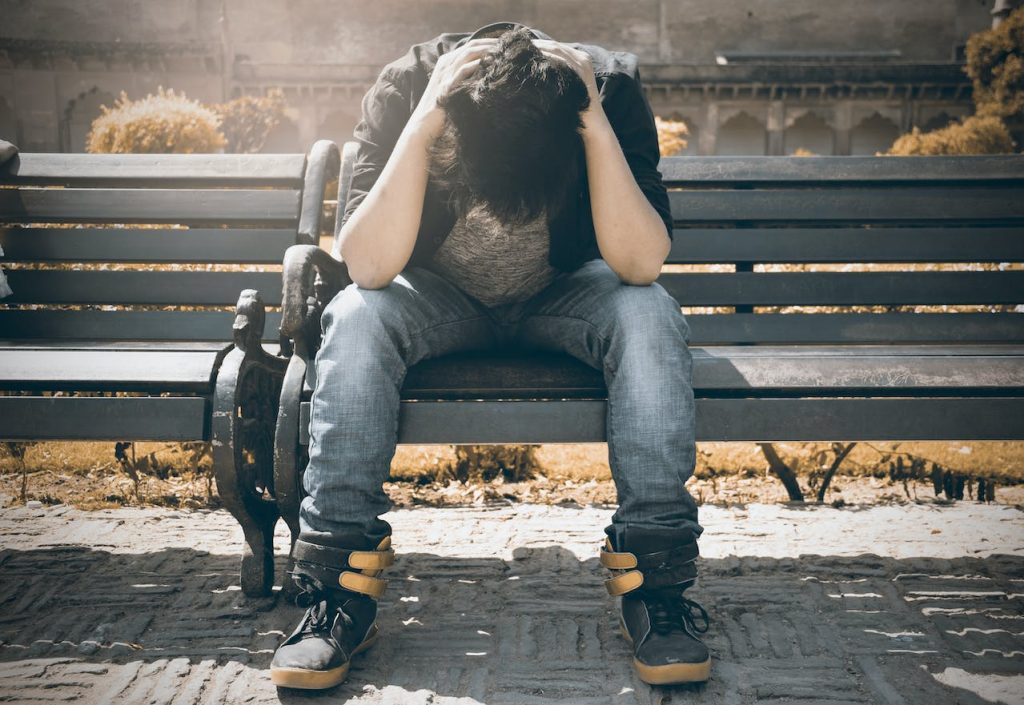
Rising health care prices in the US are leading employers outside the health care sector to lay off employees, according to a new study co-authored by a Yale economist.
The study, published June 24 as a working paper by the National Bureau of Economic Research (NBER), found that when health care prices increased, non-health care employers responded by reducing their payroll and cutting the jobs of middle-class workers. For the average county, a 1% increase in health care prices would reduce aggregate income in the area by approximately $8 million annually.
The study was conducted by a team of leading economists from Yale, the University of Chicago, the University of Wisconsin-Madison, Harvard University, the US Internal Revenue Service (IRS), and the US Department of the Treasury.
“When health care prices go up, jobs outside the health care sector go down,” said Zack Cooper, an associate professor of health policy and of economics at Yale University. “It’s broadly understood that employer-sponsored health insurance creates a link between health care markets and labour markets. Our research shows that middle- and lower-income workers are shouldering rising health care prices, and in many cases, it’s costing them their jobs. Bottom line: Rising health care costs are increasing economic inequality.”
“Rising prices are hurting the employment outcomes for workers who never went to the hospital.”
Zack Cooper, Yale economist
To better understand how rising health care prices affect labour market outcomes, the researchers brought together insurance claims data on approximately a third of adults with employer-sponsored insurance, health insurance premium data from the US Department of Labor, and IRS data from every income tax return filed in the United States between 2008 and 2017. They then used these data to trace out how an increase in health care prices, such as a $2000 increase on a $20 000 hospital bill, flows through to health spending, insurance premiums, employer payrolls, income and unemployment in counties, and the tax revenue collected by the federal government.
“Many think that it’s insurers or employers who bear the burden of rising health care prices. We show that it’s really the workers themselves who are impacted,” said Zarek Brot-Goldberg, an assistant professor at the University of Chicago. “It’s vital to understand that rising health care prices aren’t just impacting patients. Rising prices are hurting the employment outcomes for workers who never went to the hospital.”
Hospital Mergers Raised Prices
For the new study, the authors used hospital mergers as a vehicle to assess the effect of price increases. From 2000 to 2020, there were over 1000 hospital mergers among the approximately 5000 US hospitals. In past work, the authors found that approximately 20% of hospital mergers should have been expected to raise prices by lessening competition, according to merger guidelines from the Department of Justice and the Federal Trade Commission. These mergers, on average, raised prices by 5%.
“We can use our analysis to estimate the effect of hospital mergers,” said Stuart Craig, an assistant professor at the University of Wisconsin-Madison Business School. “Our results show that a hospital merger that raised prices by 5% would result in $32 million in lost wages, 203 lost jobs, a $6.8 million reduction in federal tax revenue, and a death from suicide or overdose of a worker outside the health sector.”
The study also showed that because rising health care prices leads firms to let go of workers, a knock-on effect of hospital mergers is that they lead to increases in government spending on unemployment insurance and reductions in the tax revenue collected by the federal government.
“It’s vital to point out that hospital mergers raise spending by the federal government and lower tax revenue at the same time,” said Cooper. “When prices in the US health sector rise, it’s actually a net negative for the economy. It’s leading to fewer jobs and precipitating all the consequences we associate with workers becoming unemployed.”
Source: Yale University